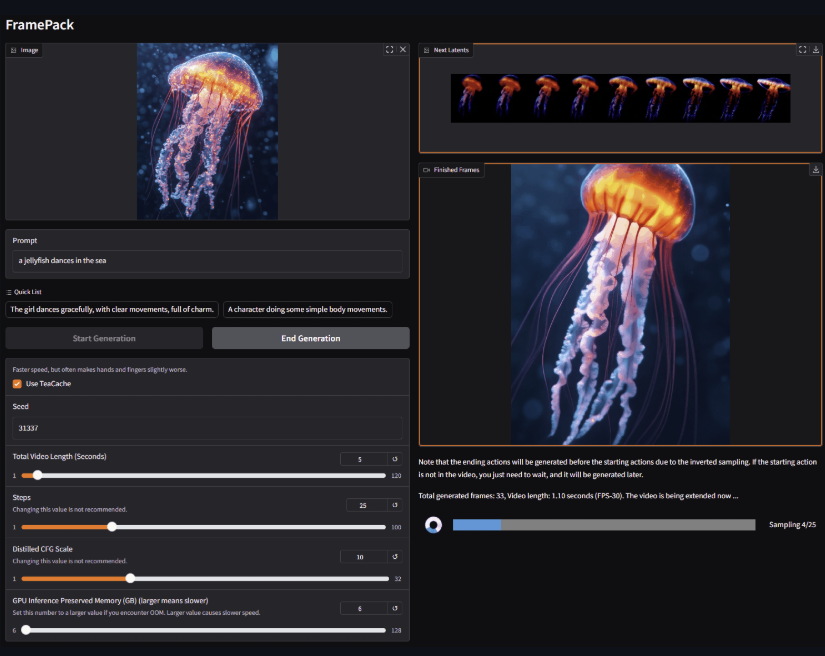
Video generation has taken a leap forward with FramePack, a groundbreaking neural network structure that redefines how we create videos using next-frame prediction models. Unlike traditional video diffusion methods that struggle with long sequences and heavy computational demands, FramePack compresses input contexts to a constant length, making video generation as intuitive as working with images. This article explores how FramePack transforms video generation, its innovative approach to handling large frame counts, and why it feels like a game-changer for creators and developers alike.
What is FramePack?
FramePack is a next-frame prediction model designed to generate videos progressively, frame by frame. At its core, it tackles a persistent challenge in video generation: managing the growing complexity of long video sequences. By compressing the context of input frames into a fixed-length representation, FramePack ensures that the computational workload remains consistent, regardless of video length. This efficiency allows even modest hardware, like laptop GPUs, to process videos with billions of parameters, such as 13B models.
Moreover, FramePack’s design draws inspiration from image diffusion techniques, making video generation feel familiar and streamlined. For developers accustomed to image-based workflows, this approach feels like a natural extension, bridging the gap between static and dynamic media.
Why Context Packing Matters
In traditional video generation, models must process an ever-increasing number of frames as a video grows longer. This leads to skyrocketing computational demands, often rendering long-form video generation impractical on standard hardware. FramePack, however, flips this paradigm. By packing the context of previous frames into a compact, constant-length format, it eliminates the need to reprocess entire sequences for each new frame.
For example, imagine generating a 10-minute video. A conventional model might struggle with the cumulative weight of thousands of frames, but FramePack maintains a steady workload, enabling smooth and scalable generation. Consequently, this innovation opens the door to creating longer, more complex videos without requiring supercomputers.
The Technical Edge of FramePack
Constant-Length Context Compression
At the heart of FramePack lies its ability to compress input frame contexts. Instead of storing and processing every frame individually, the model distills relevant information into a fixed-size representation. This technique not only reduces memory usage but also ensures that generation speed remains consistent, even for extended sequences.
To illustrate, consider a video with 1,000 frames. A typical model might need to revisit hundreds of prior frames for each prediction, slowing down as the sequence grows. FramePack, on the other hand, uses its compressed context to make predictions efficiently, regardless of the video’s length.
Scalability with Large Models
Another key point is FramePack’s compatibility with large-scale models. With 13B parameter models, it can handle massive frame counts without breaking a sweat. Surprisingly, this capability extends to modest hardware, such as laptop GPUs, making advanced video generation accessible to a broader audience. For instance, creators no longer need specialized data centers to produce high-quality, long-form content.
Training Efficiency
FramePack also shines during training. By compressing contexts, it allows for larger batch sizes, similar to those used in image diffusion training. This means developers can train models faster and with greater stability, reducing the time and resources needed to achieve optimal performance. In short, FramePack makes the training process feel more like working with images than wrestling with cumbersome video data.
FramePack vs. Traditional Video Diffusion
Video diffusion models, while powerful, often feel clunky when handling long sequences. They require significant computational resources, and their performance can degrade as videos grow in length. FramePack, by contrast, offers a refreshing alternative. Its image-like workflow simplifies the generation process, making it more intuitive and efficient.
For example, in traditional video diffusion, generating a single frame might involve processing a large chunk of the preceding sequence. FramePack streamlines this by relying on its compressed context, reducing both time and resource demands. As a result, creators can focus on crafting compelling content rather than battling technical limitations.
Real-World Applications
The implications of FramePack’s efficiency are vast. Here are a few ways it’s poised to transform industries:
- Content Creation: Filmmakers and animators can generate extended scenes or entire films with minimal hardware, democratizing access to high-quality video production.
- Gaming: Game developers can create dynamic, real-time cutscenes or procedural environments, enhancing player immersion without taxing system resources.
- Education and Training: FramePack enables the creation of detailed instructional videos or simulations, making complex concepts more accessible through visual storytelling.
- Advertising: Marketers can produce tailored, high-quality video ads quickly, adapting to trends without delays caused by resource-intensive workflows.
In each case, FramePack’s ability to handle large frame counts efficiently translates to faster production times and greater creative freedom.
Challenges and Future Directions
While FramePack is a significant step forward, it’s not without challenges. For one, compressing context into a constant length requires careful design to avoid losing critical details. If the compression is too aggressive, the model might miss subtle nuances, impacting video quality. Developers must strike a balance between efficiency and fidelity, a task that ongoing research continues to refine.
Additionally, while FramePack excels with large models, optimizing it for smaller, resource-constrained devices remains a priority. Future iterations could focus on lightweight versions that maintain performance while further reducing hardware demands.
Looking ahead, FramePack’s approach could inspire new architectures for other sequential data tasks, such as audio generation or time-series prediction. By applying context packing to these domains, researchers might unlock similar efficiencies, broadening the impact of this innovation.
How FramePack Feels Like Image Diffusion
One of FramePack’s most compelling features is how it mirrors the simplicity of image diffusion. Developers familiar with generating static images will find FramePack’s workflow intuitive. The constant-length context eliminates the need to manage sprawling frame sequences, making video generation feel like tweaking a single, cohesive image.
For instance, just as an image diffusion model refines a static scene iteratively, FramePack refines a video frame by frame, using its compressed context as a guide. This similarity not only lowers the learning curve but also makes FramePack a versatile tool for creators transitioning from image to video work.
Why FramePack is a Game-Changer
FramePack’s ability to process vast frame counts on modest hardware is a democratizing force. Previously, high-quality video generation was the domain of studios with access to powerful servers. Now, individual creators, small businesses, and hobbyists can experiment with advanced models, leveling the playing field.
Moreover, its training efficiency accelerates innovation. Researchers can iterate faster, testing new ideas without waiting days for models to train. This speed could lead to rapid advancements in video generation, pushing the boundaries of what’s possible.
Finally, FramePack’s image-like workflow bridges a critical gap. By making video generation as approachable as image creation, it invites a wider community to explore and innovate, fostering a new wave of creative and technical breakthroughs.
Conclusion
To wrap up, FramePack represents a bold step forward in video generation. Its innovative context-packing approach makes long-form video creation efficient, scalable, and accessible, all while feeling as intuitive as image diffusion. For creators, developers, and researchers, FramePack opens up a world of possibilities, from crafting cinematic masterpieces to building immersive gaming experiences. As we move forward, the impact of FramePack will likely extend beyond video, inspiring new ways to handle sequential data across industries.
Leave a Reply